The Role of Machine Learning in Autonomous Vehicles
Machine learning (ML) is the engine driving the rapid evolution of autonomous vehicles. By enabling self-driving cars to process data, make decisions, and adapt to dynamic environments, machine learning has transformed the way we think about transportation. This cutting-edge technology is not just futuristic but practical, setting the stage for safer and smarter roads. In this blog, we’ll explore how machine learning powers autonomous vehicles, the critical technologies involved, and the road ahead for artificial intelligence in cars.
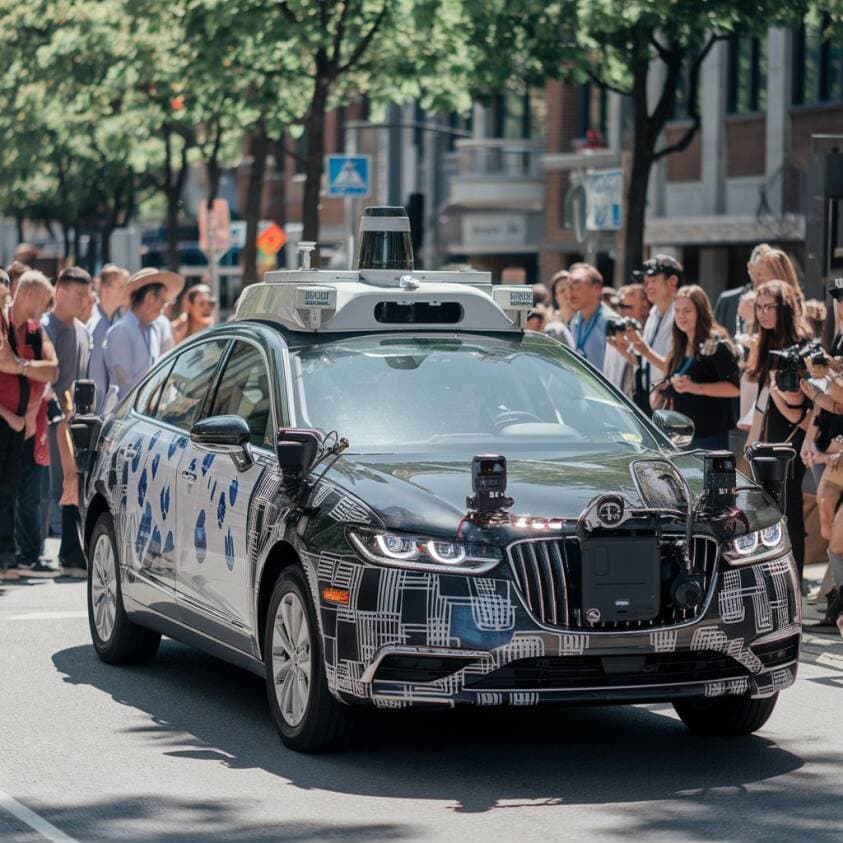
Machine learning (ML) is the engine driving the rapid evolution of autonomous vehicles. By enabling self-driving cars to process data, make decisions, and adapt to dynamic environments, machine learning has transformed the way we think about transportation. This cutting-edge technology is not just futuristic but practical, setting the stage for safer and smarter roads.
In this blog, we’ll explore how machine learning powers autonomous vehicles, the critical technologies involved, and the road ahead for artificial intelligence in cars.
How Machine Learning Empowers Autonomous Vehicles
1. Perception and Environment Mapping
Machine learning allows autonomous vehicles to interpret the world around them by processing data from multiple sources, including cameras, lidar, radar, and ultrasonic sensors.
Key Applications:
-
Object detection: Identifying cars, pedestrians, and obstacles.
-
Lane recognition: Ensuring the vehicle stays within its designated path.
-
Traffic signal detection: Understanding when to stop or go.
ML models, particularly those using neural networks, are trained on vast datasets to recognize patterns and classify objects accurately.
2. Decision-Making and Navigation
Machine learning drives the decision-making capabilities of self-driving cars. Whether it’s determining the safest way to merge onto a highway or avoiding a sudden obstacle, ML algorithms analyze real-time data to make critical choices.
Key Functions:
-
Predicting the actions of nearby vehicles and pedestrians.
-
Selecting optimal routes based on traffic and road conditions.
-
Ensuring smooth transitions between different driving modes.
Reinforcement learning—a technique where algorithms learn by trial and error—plays a vital role in improving these decision-making processes.
3. Continuous Learning and Adaptation
Autonomous vehicles rely on machine learning for continuous improvement. Each journey provides new data that refines the vehicle's ability to handle diverse scenarios.
For example, self-driving cars operating in urban environments collect information on unusual traffic patterns, helping their systems adapt for future trips. This process, often called "fleet learning," allows entire networks of autonomous vehicles to benefit from shared data.
CarzOnWheel Blogs are your go-to for everything cars! Get the latest reviews, expert opinions, and updates on the hottest trends in the automotive world. Plus, don’t miss out on our exclusive offers on top car models and services.
The Role of AI in Automotive Safety
Artificial intelligence in cars, powered by ML, significantly enhances safety by reducing human errors, the leading cause of accidents. Features like adaptive cruise control, emergency braking, and lane-keeping assistance stem from advancements in machine learning.
ML’s predictive capabilities enable vehicles to anticipate and respond to potential hazards more quickly than a human driver.
Benefits of Machine Learning in Autonomous Vehicles
-
Enhanced Efficiency:
-
Optimized fuel consumption or battery usage through intelligent route planning.
-
Improved Safety:
-
Reduced risk of accidents through real-time hazard detection.
-
Scalability:
-
Ability to deploy ML models across large fleets for consistent performance.
-
Reduced Traffic Congestion:
-
Smarter navigation systems minimize travel time and alleviate congestion.
Challenges in Machine Learning for Self-Driving Cars
1. Data Dependency
Autonomous vehicles require vast amounts of high-quality data for training ML models. Gathering and labeling this data is both time-consuming and costly.
2. Edge Cases
Handling rare and unpredictable scenarios—like animals crossing the road or sudden weather changes—remains a significant challenge for ML systems.
3. Ethical Dilemmas
Machine learning must address moral questions, such as how to prioritize safety in unavoidable collision scenarios.
4. Computational Requirements
The processing power needed for real-time decision-making is immense, making hardware development a crucial aspect of autonomous technology.
Key Technologies in Machine Learning for Self-Driving Cars
-
Deep Learning:
-
Neural networks process images and sensor data for object detection and classification.
-
Reinforcement Learning:
-
Models improve decision-making by simulating various driving scenarios.
-
Sensor Fusion:
-
Combines data from multiple sensors for a comprehensive view of the vehicle’s surroundings.
-
Natural Language Processing (NLP):
-
Powers voice-controlled systems in autonomous vehicles for a seamless user experience.
Future Trends in Machine Learning and Autonomous Vehicles
The future of machine learning in autonomous vehicles is promising, with several trends on the horizon:
-
Edge AI: Processing data locally on the vehicle to reduce reliance on cloud-based systems.
-
Improved Generalization: Models capable of adapting to diverse driving environments worldwide.
-
Integration with V2X Communication: Machine learning will work alongside vehicle-to-everything (V2X) technology to improve situational awareness and coordination.
Conclusion
Machine learning is the cornerstone of autonomous vehicles, driving advancements in perception, decision-making, and navigation. While challenges remain, the ongoing development of AI and ML technologies ensures that self-driving cars will continue to improve, making roads safer and travel more efficient.
As the role of machine learning in autonomous driving evolves, its impact will extend far beyond the automotive industry, reshaping transportation as we know it.